Speaker's bio
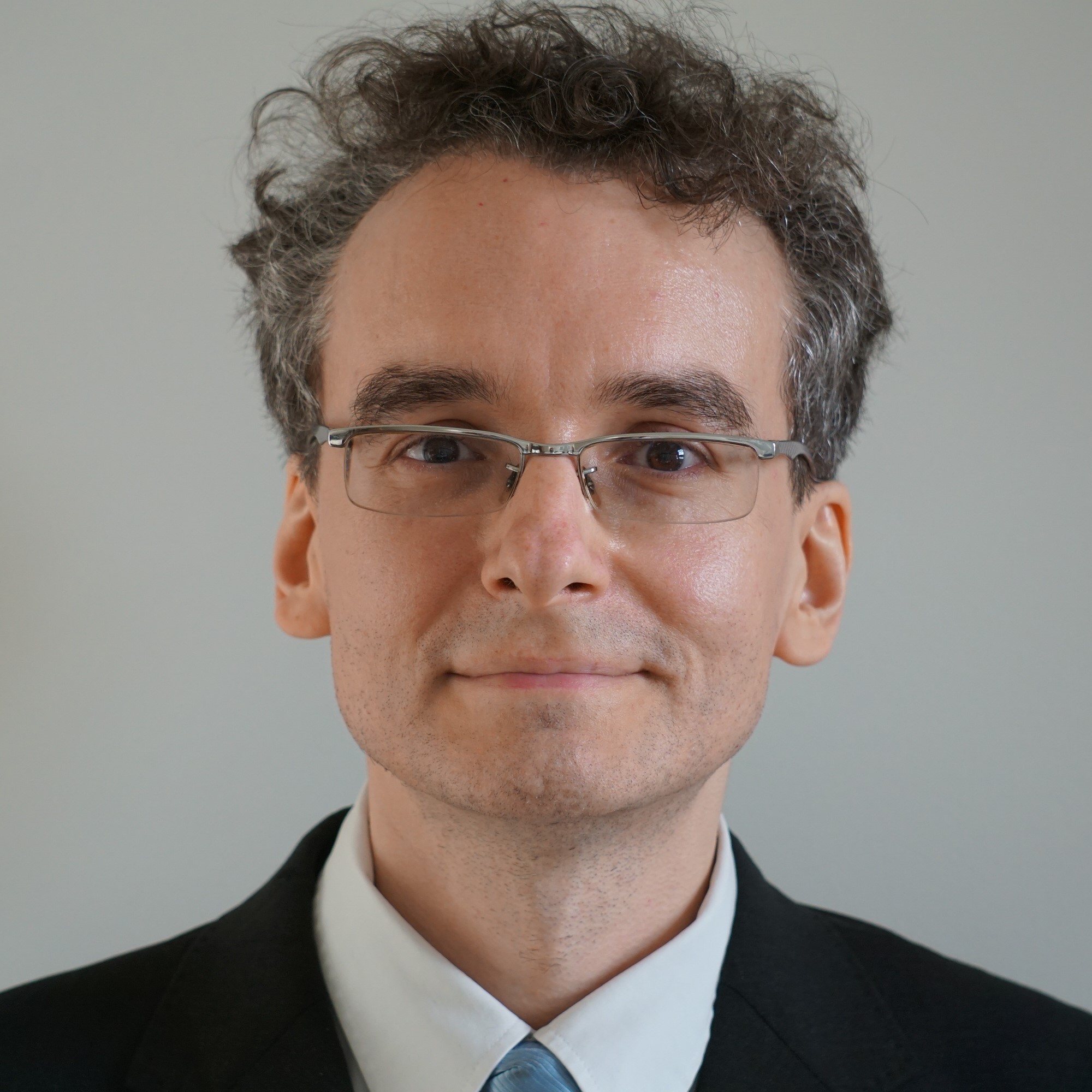
Attila
Gáspár
(Morgan Stanley)
Attila Gaspar is a Vice President at Morgan Stanley in the Budapest Regulatory Modeling team. In his current role, he manages and works on several model development projects for CCAR, focusing on forecasting and stress testing firm-specific variables. He has 15+ years of experience in various areas of modeling, including forecasting micro data like net revenue and balance sheets but also macro data like GDP and other national account aggregates. He is also regularly mentoring junior model developers and holds knowledge-sharing sessions.
Prior to joining Morgan Stanley in 2019, Attila was working in the public sector in various institutions, including the Ministry of Finance, Institute for Public Policy Research, and Hungarian Central Statistical Office. He was developing macroeconometric models and analyzing various macroeconomic data. Attila has been teaching quantitative and macroeconomic courses as an external lecturer in various universities, including ELTE and Budapest Business School. Currently, he is teaching at Corvinus University. He is conducting research, which includes writing and reviewing papers, also presenting at conferences; his core area of interest is Growth Econometrics.
Attila holds a Ph.D. degree in Economics from the University of Debrecen, an MSc in Financial Engineering from WorldQuant University, an MA in Economics from Corvinus University, and a BA in International Economics from Budapest Business School. Attila took 30+ additional courses from various institutions like IMF, Joint Vienna Institution, Central European University, and Eurostat.
Opportunities and Challenges of Advanced Forecasting Methods
Various shocks and uncertainties raise new challenges of modelling in industry practice: advanced methods driven by academic research can potentially solve for these challenges. For instance, firm specific variables can behave very differently in various baseline and stress scenarios, hence, regime switching approaches may lead to smaller forecast errors compared to standard techniques. Besides that, there are also various other areas where new academic research can bring in new ideas into industry. For instance, statistical tests have to be adjusted due to small sample size, where the critical values are taken from academic papers. In this presentation a few examples and challenges are shown based on some findings from Morgan Stanley Finance. The regulatory modelling team is exploring various methods based on econometric and machine learning techniques to improve the forecasting power of models, and on the top of that the team works closely with universities. Hence, there are several approaches supporting synergies across industry and academic practice within the team.