Generating synthetic data using Variational Autoencoders
Team:
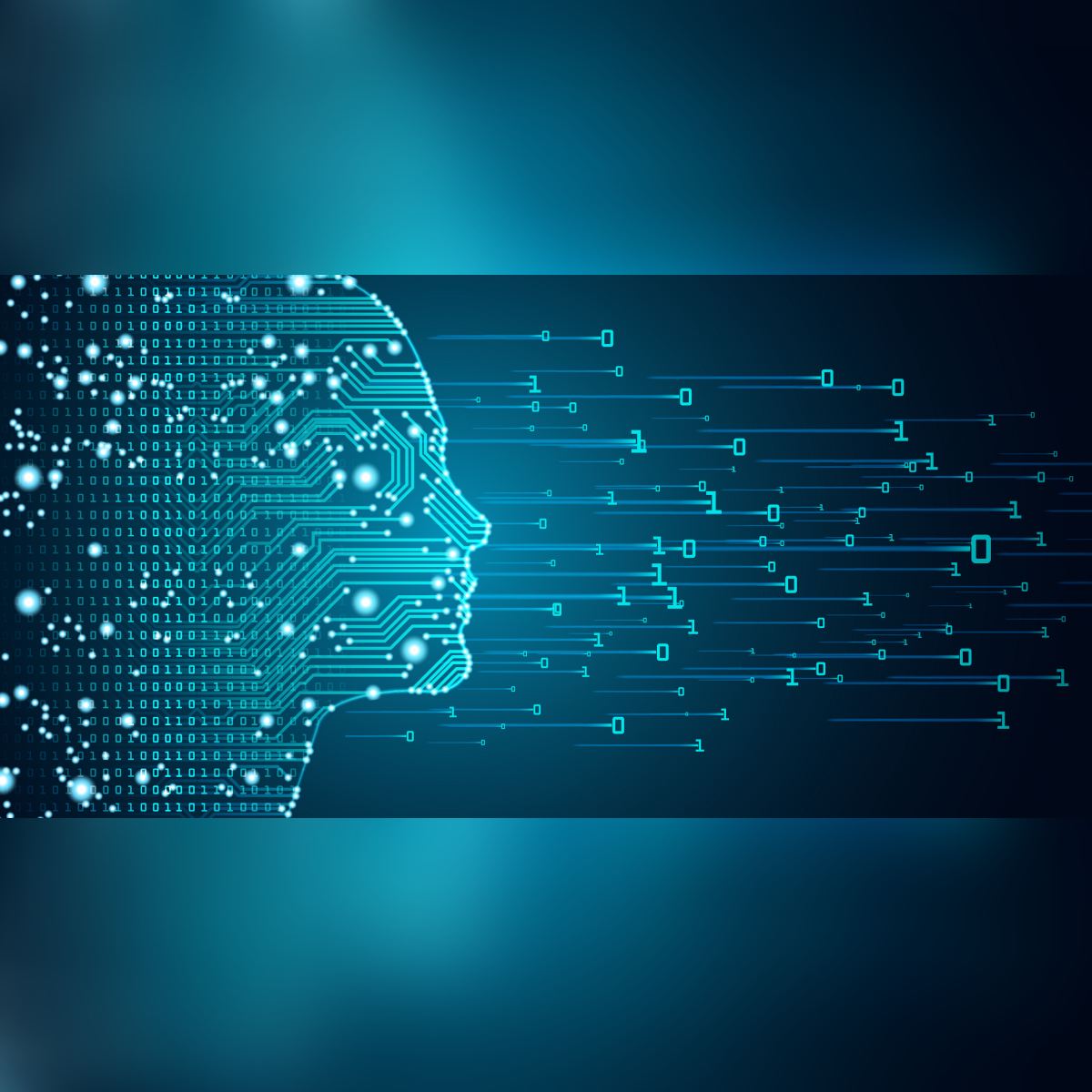
In this project, students will delve into the innovative world of machine learning and explore the capabilities of Variational Autoencoders (VAEs) to create synthetic data. VAEs are cutting-edge generative models that excel in capturing complex data distributions and generating new samples that closely resemble the original data. Through hands-on experience, participants will develop a deep understanding of VAEs' architecture, training methodologies, and their role in data augmentation. By mastering this technique, students will contribute to solving real-world challenges in various fields where data scarcity is a concern. Join us in this project to unlock the potential of synthetic data generation using VAEs and make a meaningful impact on applications ranging from financial market modeling to asset pricing to anomaly detection and beyond.
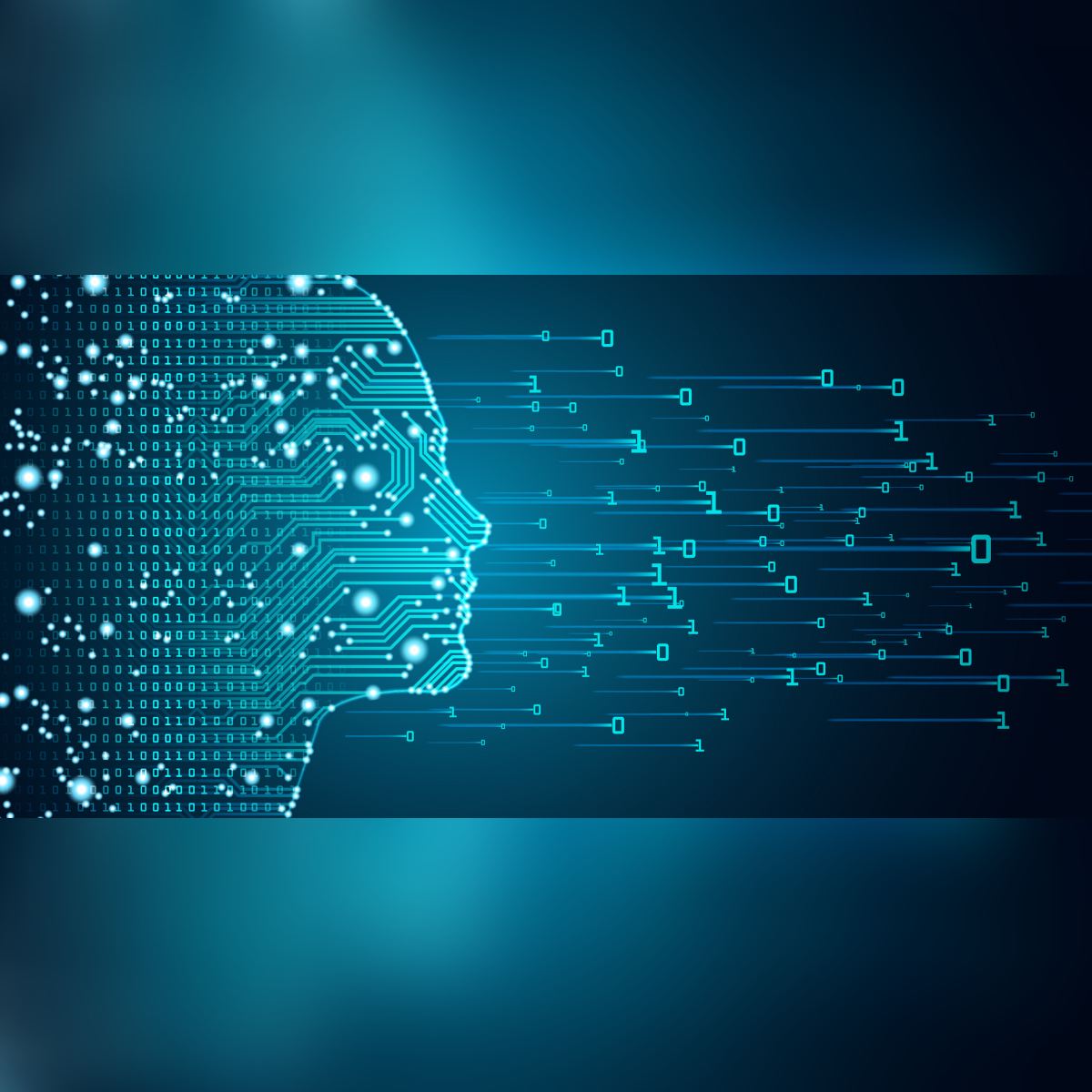
In this project, students will delve into the innovative world of machine learning and explore the capabilities of Variational Autoencoders (VAEs) to create synthetic data. VAEs are cutting-edge generative models that excel in capturing complex data distributions and generating new samples that closely resemble the original data. Through hands-on experience, participants will develop a deep understanding of VAEs' architecture, training methodologies, and their role in data augmentation. By mastering this technique, students will contribute to solving real-world challenges in various fields where data scarcity is a concern. Join us in this project to unlock the potential of synthetic data generation using VAEs and make a meaningful impact on applications ranging from financial market modeling to asset pricing to anomaly detection and beyond.