Generating synthetic financial data using GANs
Team:
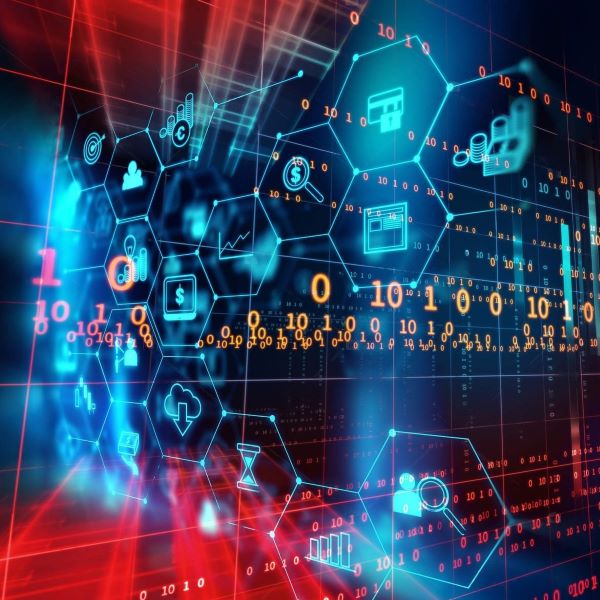
Generative Adversarial Networks (GANs) are powerful AI algorithms capable of creating artificial data closely mimicking the statistical properties of actual data. GANs consist of a generator network that generates the data and a discriminator network that tries to distinguish between the real and the artificial. Students will explore GAN fundamentals, implement GANs in Python TensorFlow and experiment with various architectures, fine-tune parameters to produce high-quality synthetic financial time series data. This opportunity offers hands-on experience at the intersection of AI and quantitative finance, enhancing coding and data manipulation skills and the understanding of the statistical properties of real financial time series data.
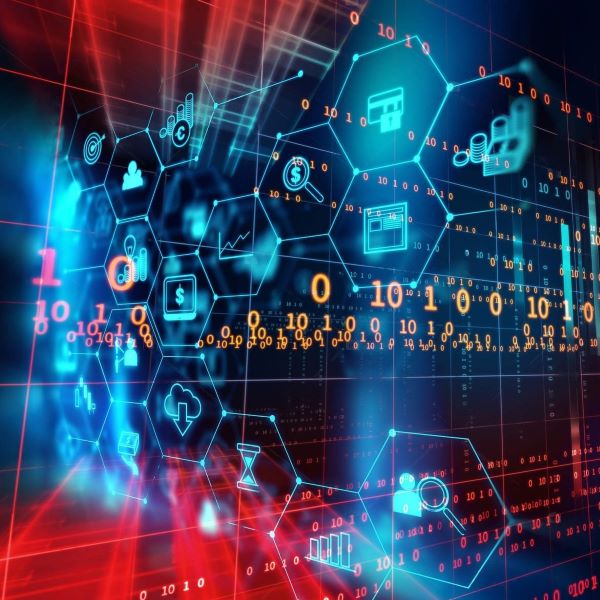
Generative Adversarial Networks (GANs) are powerful AI algorithms capable of creating artificial data closely mimicking the statistical properties of actual data. GANs consist of a generator network that generates the data and a discriminator network that tries to distinguish between the real and the artificial. Students will explore GAN fundamentals, implement GANs in Python TensorFlow and experiment with various architectures, fine-tune parameters to produce high-quality synthetic financial time series data. This opportunity offers hands-on experience at the intersection of AI and quantitative finance, enhancing coding and data manipulation skills and the understanding of the statistical properties of real financial time series data.